Turing Talk: digital twins, communicating the complexity of AI projects, and credit for research software engineers
This year’s Turing Talk was given by Mark Girolami, an academic statistician and the Sir Kirby Laing Professor of Civil Engineering at the University of Cambridge. Mark discussed the idea of digital twins, traced their history and discussed some of the opportunities and challenges ahead. The talk ticked all the boxes that an event like this is supposed to tick - an interesting contemporary topic made accessible to a non-expert audience who wanted to be inspired and learn something new.
The market for digital twins (complex digital models of the real world) is enormous and was valued at USD $3.8bn in 2019 and is expected to reach USD $35.8bn by 2025. Half of all large industrial companies are predicted to be using digital twins in some form by 2021. Of course, governments want their own digital twins to model the impact of policy changes but, given the unpredictability of human behaviour, they’ll take a ‘bit’ longer to develop. Some examples of the amazing work done by the Data-centric engineering programme shown during the talk can be found on the The Alan Turing Institute's website.
Couple of thoughts I had following the talk:
1: Communicating the complexity of AI projects
Mark gave examples of various analogue and digital twins and how they have developed over time. At the end of the talk a lady in the audience asked, when would she have her own digital twin who could tell her that she was likely to catch a cold next week? I thought Mark’s answer was great. Paraphrasing, he said, do you remember the example of the 1980s man with the moustache and CAD system? The work it took to get from those kinds of systems (advances in computing technology, new forms of mathematics, new kinds of algorithms, etc.) to what we have now is about how much work is needed to get from where we are now to being able to predict that you’re going to get a cold.
I love these kinds of answers. A while back I was discussing a potential use of AI tech in publishing with Phil from Scholarcy and he said that the technology needed was at least 30 years away. It was such an easy, informative and non-patronising answer. If you try to explain to me why a certain AI project is complex I will almost certainly get lost in the details. If you tell me it’s a very hard thing to do I will almost automatically ask why is it so hard (although I probably don’t really want to know the details), but if you tell me the technology needed is XX years away from being able to solve that kind of problem then I can quickly understand and the conversation on.
This reminded me of Elisabeth Bik’s problem with people ‘helpfully’ suggesting that AI could help detect fraudulent reserch images. If the AI community could quantify how hard this kind of problem is, would it help? Jigsaw’s Assembler project which aims to help journalists spot image manipulation have just published the learnings from their Alpha testing. They do a good job of explaining some of the challenges this kind of work entails but, as a non expert, it’s still difficult to get a sense of how challenging these problems are or how far away they are from a solution. Is it the kind of problem their team might be able to work out in six months, a year or two, or is it 5-10 years away? If you’re not working in the field it’s very hard to separate hype from reality with AI technologies.
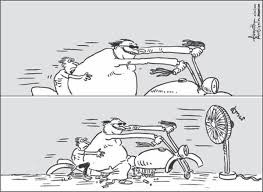
2: Software and research assessment
Sitting in the Alan Turing lecture theatre following registration in the Tommy Flowers room looking at examples of cutting edge data/software engineering reminded me of a conversation with a friend about academic promotion. She was an external assessor for two computer science academics who wanted a promotion. Simplifying, one was based in a prestigious UK university, he was running the department and had first author papers in the top computer science journals. She said it was an easy decision to recommend that the person be promoted. The second decision was far more difficult, this researcher was based at a middle-eastern university but his only first author papers were published in local computer science conference proceedings which she couldn’t access. He was listed as an author in a number of papers published in various medical journals but his contribution was unclear (he probably built the models upon which the medical research was based). The university promotion form was designed to highlight only research papers published and not other forms of contribution. She said she had no idea what to recommend because of the lack of information. I am guessing that this kind of problem is only going to increase for the highly skilled and inventive ‘data engineers’ who build and develop these huge data sets, models and visualisations but who are very unlikely to be the first author in the resulting research papers. (More about this on the Software Sustainability Institute blog). In theory CRedIT should help but I wonder, like Elizabeth Gadd, if some roles might be prized above others and if software contributors might end up receiving less credit rather than more with this system.