Brian Nosek’s talk about Open Science was suitably inspirational and visionary for the believers but perhaps a tad too evangelical for the merely curious. [Slides here]. Two thoughts stuck with me after the talk:
There are huge opportunities for publishers who need to find alternative sources of revenue pronto
Where is Open Science on the hype cycle?
Opportunities
Open Science principles and solutions have been around for a while and have been agreed and codified by Open Science’s early adopters so all that needs to be done now, simplifying Brian’s lecture just a bit :-), is some culture change to move Open Science practices into the mainstream.
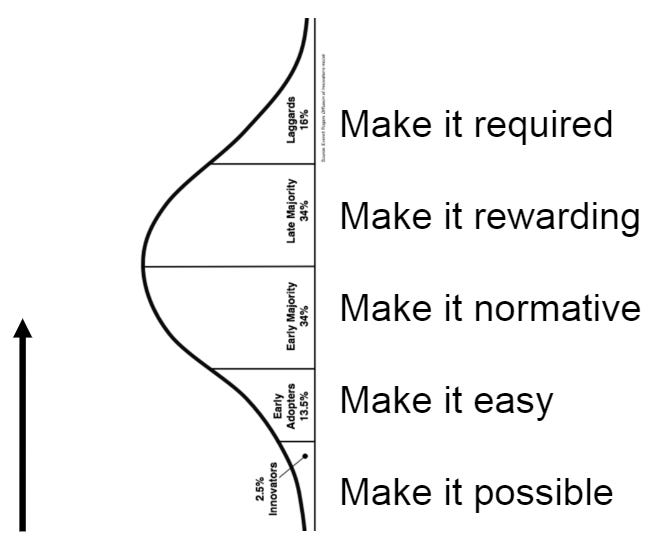
Brian talked about how the COS are encouraging the community to use badges, preprints, registered reports, transparency policies, etc. to make culture change happen. You can’t fault the approach - it’s well thought out textbook change management stuff. However, Brian didn’t show the other version of this diagram, the one which shows the chasm a new technology has to cross before the early majority will adopt which might prove to be quite a tough challenge for Open Science.
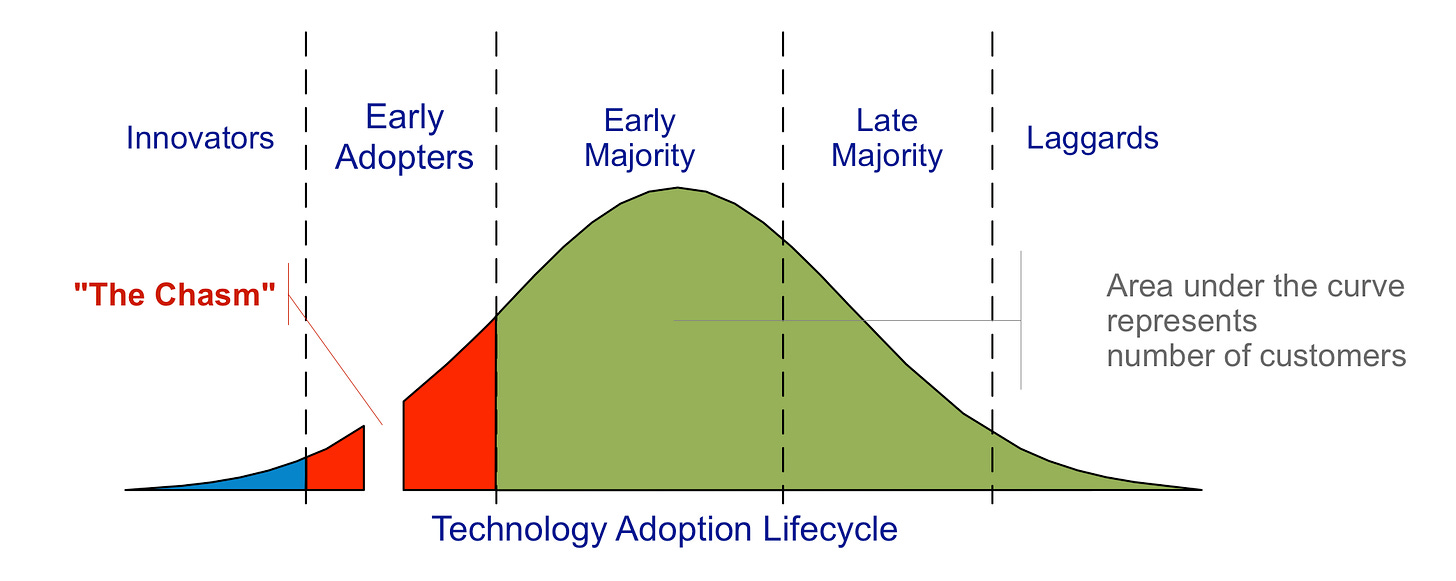
Making Open Science principles mainstream is largely a culture change problem, operationalising Open Science is more of a technology problem and there are plenty of opportunities for new products and services. A few [uninformed] ideas:
Allocating OA funds. Given the way transformative deals and Gold OA publishing models are being adopted I am fairly sure this is going to become an organizational nightmare. It’s pretty unlikely a research intensive university will have enough funds to allow researchers to publish everything they want so the university will need transparent ways of allocating publication funds. Tricky nut to crack for Institutions needing to fund science, medicine, social science and arts publications out of the same pot of money [more on this here].
Managing and commercialising data. There are services that help researchers manage lab data, trial data, etc. There are services that help researchers store and manage datasets but these tend to be static repository systems. There are lots of services that manage rights. Commercialising, licensing, monitoring usage, restricting access to certain groups and ensuring ethical use of data is where things could get really interesting and where there must be many opportunities for new API based services.
Ensuring data integrity. There’s a training and a technical element to this. No doubt we’ll see a large number of courses and other initiatives being developed to train researchers in best practices. On the technical side, how much trust will researchers place in someone else’s data? I suspect automated technical checks to ensure there's nothing obviously amiss in the data (and maybe another badge to certify this) will no doubt be welcome by people wanting to reuse data from people they may not know or trust. These kinds of tools already exist but they haven’t been specifically designed for research data/to work in a university environment.
Registered reports and peer review. Registered reports require peer review before doing the research and, if following a traditional model, before publication. That’s a lot of peer review in a system that’s already under strain. I suspect more standardised (cookbook) methodologies will appear particularly in the social sciences to reduce the burden. For taught postgraduate courses I am sure most students would welcome being given a workbook that takes them through the design of their study (not like the research methods textbooks which are hopelessly vague about you actually have to do). For a social science survey, the tool could collect the survey data, automatically work survey response rates and their significance, generate graphs and include a robust way of reporting on if results have been excluded to achieve a better result (survey tools like SurveyMonkey kind of do this now but add a bit more support/prompts for researchers at the beginning and end of the process and you would have an awesome research tool).
New formats and automation. If researchers are using standardised tools to conduct their research there seems little reason why publishers can’t automate a lot more of the publication process. Again for taught postgrad courses, build the tool that collects and interprets the data and then allow students to publish their dissertation as a short formulaic kind of paper in a dedicated journal with minimal fuss using data already in the system, especially if it’s a replication study.
The “trough of disillusionment”
My second thought was about where Open Science fits on Gartner‘s Hype Cycle:
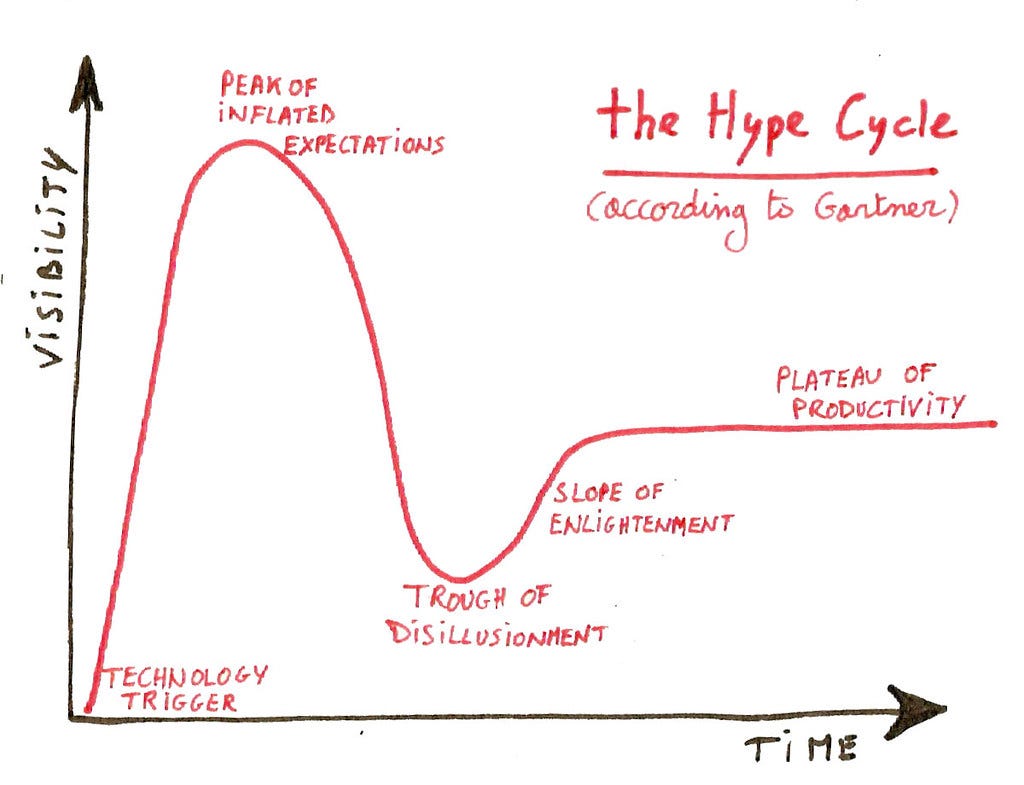
I don’t think Open Science is close to reaching the ‘peak of inflated expectations’ just yet but I think it’s coming. Some of the benefits of Open Science initiatives do appear a little bit too good to be true. It seems inevitable that some of the early adopters will get frustrated if the system around them isn’t changing as quickly as they had hoped, or if following open science principles doesn’t bring career benefits, or their data gets combined with other data to create something they would never want to put their name to, or that there are still too many niggles which need to be worked out. For example, two of the more interesting questions from the audience were about how to deal with fast moving fields in which the methods you put in your registered report are out of date when you come to do the analysis? And how does Open Science work in research areas where patents are the norm? For some, I suspect there will also be huge disappointment if the revolution is driven by large commercial players rather than scholar-led not-for-profits!
Many thanks to Brian Nosek, co-Founder and Executive Director of the Center for Open Science for speaking and to Professor David Price and team for organising an excellent lecture.